AI chatbots could converse all day without crashing, new research finds
Researchers at MIT have found a solution to the problem of AI chatbots’ deteriorating conversations, enabling them to maintain nonstop […] The post AI chatbots could converse all day without crashing, new research finds appeared first on ReadWrite.
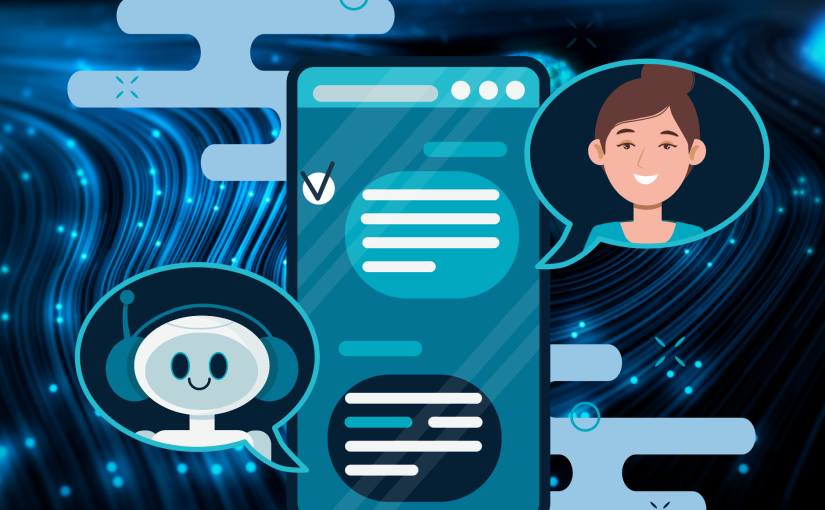
Researchers at MIT have found a solution to the problem of AI chatbots’ deteriorating conversations, enabling them to maintain nonstop conversations without crashing or slowing down.
When users continuously converse with chatbots like ChatGPT, the large language models powering the technology begin to collapse, leading to communication issues. At times, they can even hallucinate facts.
However, some researchers have identified the root cause and discovered a way to allow conversations to flow without the need to restart the software.
Their approach modifies the key-value cache, essentially the conversation memory central to many large language models. In specific methods, when the cache exceeds its capacity, it ejects the earliest data entries, which can lead to the model’s failure. However, by preserving these initial data points in its memory, they were able to push the chatbot to keep engaging without any significant issues.
Boom!
A new technique named "StreamingLLM" can handle infinite text input without any drop in accuracy by using key tokens that guide the model's decisions and caching recent tokens.
The result: 22x faster inference.https://t.co/RDeTUZ6up6
— Brian Roemmele (@BrianRoemmele) October 3, 2023
By using a technique known as StreamingLLM, the researchers were able to ensure the model stayed efficient even during conversations that extended beyond four million words. Compared to another approach that prevents crashes by frequently re-evaluating portions of previous conversations, StreamingLLM proved to be over 22 times quicker.
As a result, this could help chatbots sustain lengthy conversations without the need for constant reboots, which means that the AI assistants are far more effective for activities such as copywriting, editing, or code generation.
Why are AI chatbots crashing?
Large language models transform user queries into token representations, using an attention mechanism to generate new text by assessing how these tokens relate to each other within an “attention map.”
This process, crucial for producing human-like text, relies on storing recent tokens in a ‘KV Cache.’ However, the cache’s capacity limitations and the subsequent massive size of the attention map can slow down computations and degrade performance when the cache overflows, as seen when encoding complex documents like academic papers.
Researchers have attempted to address these issues with a “sliding cache” strategy, which replaces the oldest tokens with new ones, though this often results in a significant drop in text quality as soon as tokens are removed.
A new approach detailed in the paper suggests keeping the first token in the cache to maintain model performance, even when the cache limit is surpassed. This counterintuitive strategy is effective despite the seemingly unrelated nature of the first and last words in extensive texts and books, leading to discoveries about the underlying reasons for this phenomenon. It offers insights into improving large language model efficiency.
The lead author of the StreamingLLM paper, graduate student Guangxuan Xiao, said, “Now, with this method, we can persistently deploy these large language models. We could use these chatbots in some new applications by making a chatbot that we can always chat with and that can always respond to us based on our recent conversations.”
Feeling incredibly excited and proud that StreamingLLM made it to MIT's homepage and has been accepted by ICLR 2024! Can't wait to show it in Vienna!
What's Your Reaction?
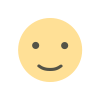
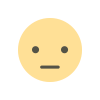

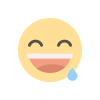


